DEEP LEARNING FOR PREDICTIVE ANALYTICS IN HEALTHCARE
Willie Bee Tingba Jr.
MEng, Information Systems Engineering Cyprus International University
Head of Department
Innovation and Information Technology
Center For Environmental and Public Health Research
(CEPRES INTERNATIONAL UNIVERSITY)
INTRODUCTION
Since hospitalization is the most common cause of costs and does not guarantee life safety due to improper analysis and prediction, health care is currently the main contributor to the economic crisis. As a result, a new, more efficient health system with cutting-edge technology and administration would offer a wealth of data that is vital for improving the real-world healthcare system.
The definitions of big data are continually changing, even though the notion is not new. The important description of the collection of components as quantity and pace, along with the kind and complication by the numerous definitions makes it possible for a person to investigate, create, and discover new hardware and software appliances to effectively maintain, examine, and envisage the data.
To comprehend and predict diseases that call for an accumulated strategy where the understanding and formalized data arising from such high amounts of data sets are managed to gather from both clinical and non-clinical sources, new technologies have emerged that make it possible to capture the enormous amount of information related to health care about each patient over time. Predictive analytics is a sophisticated form of analytics that has been increasingly popular in the big data associated with health services.
The method of data mining is used to forecast future events, and the projections are then used to provide helpful direction. Data engineering is a radical subset of data mining that goes beyond data mining and predicts probabilities or existences based on the data that is already accessible (Dr. S. Smys, 2019). The two core concepts of predictive analytics, which includes numerous statistical and analytical techniques, are categorization and extrapolation. Using the updated data as a starting point, the learning phase develops the following learning model to forecast the outcomes:
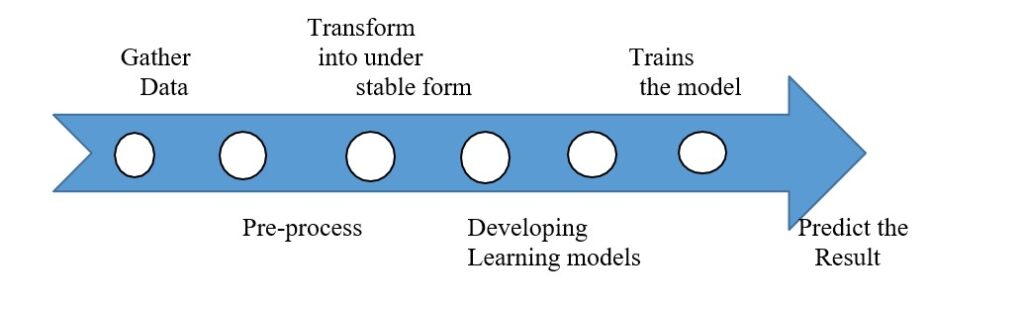
Procedure of Prediction
Predictive analytics, which is only focused on forecasting probabilities and trends, establishes a most likely future course of an event or the probability of specific current events. To automatically assess a sizable amount of data comprising various factors, it employs techniques like text mining, neural networks, decision analytics, decision trees, genetic algorithms, regression modeling, and hypothesis testing, among others.
The attribute known as the predictor, which would be frequently assessed for an individual or an entire company to forecast future events, dangers, and opportunities, is the key component of predictive analytics. Here are the first three basic techniques used in predictive analytics.
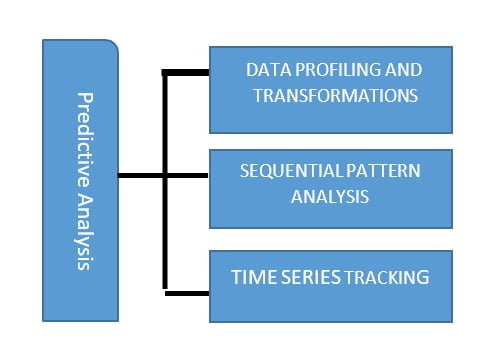
Background of Study
Deep learning predictive analysis in health obtained traction in the 1990s when HIV/AIDs and other diseases such as Alzheimer’s began to increase with little knowledge on how to diagnose, treat and cure patients of these menacing diseases. Health experts begin to think of better ways of using artificial intelligence in health equipment to deal with these. More investments in this field occurred when pandemics of 2019 and 2020 such as the Ebola Virus and Coronavirus swept across West Africa and the world killing millions and decapitating many with no signs of cure or finding a sustained vaccine.
Given the enormous cost of delayed diagnosis and treatment, the field of healthcare is one in which prediction may be more significant than explanation (Agarwal, 2014). Although the scientific method is founded on a cycle of hypothesis development, experimentation, hypothesis testing, and inference, he contends that there are various starting points. Big data is as helpful at the hypothesis generation stage as it is during the hypothesis testing stage, if not more so. In a study that aims to forecast rather than to explain, connections between variables may be uncovered that lay the groundwork for the creation of a hypothesis that can then be put to rigorous testing.
Predictive analytics helps healthcare life sciences and providers by using several methodologies from data mining, statistics, modeling, machine learning, and artificial intelligence to produce predictions about the future. It helps healthcare companies become ready by reducing costs, accurately diagnosing diseases, increasing patient care, better-utilizing resources, and improving clinical outcomes (Nithya, 2016).
The foundation of deep learning, a key technique in big data research, is the idea of automatically mining huge amounts of data to identify patterns and extract characteristics from complex unstructured data without the aid of humans. Deep learning is essential for diagnostic applications (see https://www.techemergence.com/com-learning-in-oncology).
Deep learning approaches can find clinically significant information concealed in vast volumes of data by using pertinent clinical queries. This enables doctors to precisely examine any disease for better management, which results in better medical choices. This paper discusses the current state and future possibilities of deep learning for predictive analysis in the healthcare sector. We rapidly examine the four important factors listed below in light of the perspectives of medical researchers (Hassanien, 2019):
- The deep learning framework for healthcare predictions
- The reasons for using deep learning in the healthcare industry
- The diseases that the deep learning community is now focusing on
- The tools that allow deep learning systems to produce clinically relevant overcomes
Research Problem Statement
This study aims to examine how various sectors of the healthcare industry are benefiting from predictive analytics in light of the following:
- Clinical research and medication discovery are aided by life sciences.
- Accuracy of diagnosis – Doctors can utilize predictive algorithms to aid in making more accurate diagnoses.
- Health care providers – Benefits in clinical decision support and diagnostic assistance.
- Resource optimization – Estimates of patient admissions, bed use, and duration of study can be studied along with the use of other resources to forecast future volumes.
- Set-backs in predictive analysis in developing nations, especially Africa.
Significance of Study
This paper seeks to use deep learning to analyze health issues and predict outcomes. There have been many delays in diagnosing illnesses and treatment thereof. This has led to incapacitation and many times death of patients. There is a need to digitize healthcare through the use of predictive analytics. There will be a ton of new data that requires technology from today. Without the intervention of a person, deep learning mines massive amounts of data to digitally find patterns and extract necessary information. It must be precise and capable of offering the ideal remedy for treating diseases.
The models of predictive analytics consider the importance of deep learning frameworks over traditional machine use and approach. It also shows that there is much more to be done regarding deep learning since it uses machines with no human interaction that must be accurate in treating major sicknesses. It also identifies the advantages and limitations of deep learning predictive analytics and recommends ways to improve upon it.
Objective and Hypothesis of the Research
This study aims to demonstrate how deep learning predictive analytics in healthcare has influenced clinical activities and improved the accuracy of disease diagnosis and effective treatment. It also takes into account how it is still in its early stages in developing countries due to several problems, such as weak health systems, a lack of current technology, and a shortage of skilled medical professionals.
Infrastructure, data, analytics, and application layers are required for an analytical environment. Numerous frameworks and programs can be supported by the infrastructure layer. Genetic, eligible provider (EP), and electronic medical record (EMR) data are just a few of the data sources included in the data layer. The analytics layer must support a variety of categorization, grouping, regression, etc. methods to offer predictive analytics.
Due to the unstructured nature of healthcare data, it is possible to assess certain data sources using data analysis techniques like natural language processing. The application layer facilitates the visualization of results from prediction models. The proposed approach is motivated by clinical problems and will ultimately be applied with deep learning to support clinical practice. Clinical data gathering is the first step, and clinical decision-making is the last.
The suggested system’s phases consist of:
- Natural language processing for unstructured data improvement
- Clinical decision-making
- Clinical data production
- Deep learning data analysis to identify interesting patterns
Research Question
Many questions come to mind when looking at deep learning predictive analytics. Some of them are:
- What are the deficiencies in using traditional machines in diagnosing and treating?
- What does Deep Learning Predictive Analytics provide that eliminates time consumption?
- Will the use of deep learning automation without human involvement not lead to hitches in synthesizing data and providing relevant information for treating rare illnesses?
- As Africa and the developed world prepared to adapt to this new technology given the limitation of electricity, skilled staff with the ready knowledge and expertise?
- Given the internet glitches and cyber-attacks occurring all over the world, will there be proof of protection from cyber-attacks?
Delimitation of Study
This research focuses on employing deep learning in predictive analytics in healthcare, its successes, and its setbacks. The research gathers information from health experts, patients, major health centers, the Covid Center, and makeshift health centers using available information and research done on them and through the use of several detailed journals and books on this topic.
LITERATURE REVIEW
Based on clinical and/or nonclinical trends in the data, predictive analytics in healthcare aims to predict future health-related occurrences or outcomes. By looking at the patient’s medical history, recently created deep learning systems for pharmaceutical research have shown promise in tackling several problems with drug discovery.
These programs analyze the patient’s symptoms and test findings to deliver the best therapy available. Results from studies on treatment outcomes, medical complications, hospital readmissions, patient mortality (Tabak, Using electronic health record data to develop inpatient mortality predictive model: acute laboratory risk of mortality score, 2016), hospital readmissions (Bardhan, 2017), and other topics.
The two stages are the UKPDS risk engine: 2016 model for type II diabetes and the risk of coronary heart disease, for instance, the Framingham Heart Study (D’Agostino, 2008), the UK Prospective Diabetes Study (UKPDS), and the Lung Cancer Risk Prediction Model (Tammemägi, 2013).
The utilization of patient data that has already been obtained from clinical contexts, such as EHRs, insurance claims, and clinical registries, constitutes the second technique. Consider the examples of the readmission model and the inpatient mortality forecasting model (Tabak, Using electronic health record data to construct the inpatient mortality predictive model: acute laboratory risk of mortality score, 2017). For the following reasons, predictive healthcare analytics is crucial.
A) Decision-Making Process
Predictive analytics has proven to be a very useful tool when making medical decisions. All types of medical care, especially those for long-term diseases, result in different patient reactions. A blood test created by researchers at the University of Michigan Rogel Cancer Center can assess the efficacy of a particular treatment plan for HPV-positive throat cancer months before regular imaging scans. Thanks to this type of predictive analytics, the blood test allows medical staff to assess a patient’s response to treatment months earlier than was previously feasible.
As a result, patients are spared months of unnecessary and uncomfortable care and medical experts can modify their course of therapy more rapidly if the current one is ineffective. In general, this raises the standard of patient care. In a machine learning study, researchers used predictive analytics to assess the risk of cardiovascular disease and choose appropriate treatments. To fully understand a patient’s risk factors for chronic disease, clinicians should consider the patient’s overall health. Environmental and socioeconomic aspects are included in this.
B) Improving Patient Outcomes
An innovative strategy for enhancing patient outcomes is predictive analytics. Machine learning algorithms can be programmed to provide insight into the types of treatments that will be most effective for the current patients by looking at data and outcomes of previous patients. Predictive analytics can also be used to spot warning indications before they became serious. As the COVID-19 epidemic dominates healthcare, researchers are investing time and money in creating predictive analytic strategies to fight the virus. Since the severity of COVID-19 can differ from person to person, the healthcare sector has faced a particularly challenging situation.
C) Relief For Healthcare Workers
The use of big data analytics has improved patient care and efficiency, but managing the growing body of electronic data may be exhausting for healthcare professionals. A recent study found that reading electronic health records (EHRs) takes up 62% of a doctor’s time with each patient, with clinical data evaluation taking up the majority of that time. According to the report, an artificial intelligence system should help organize EHR data so that doctors may work more productively and give patients a better experience. Twelve gastrointestinal doctors participated in the study and used the AI-powered EHR system to compare it to their previous data review experiences.
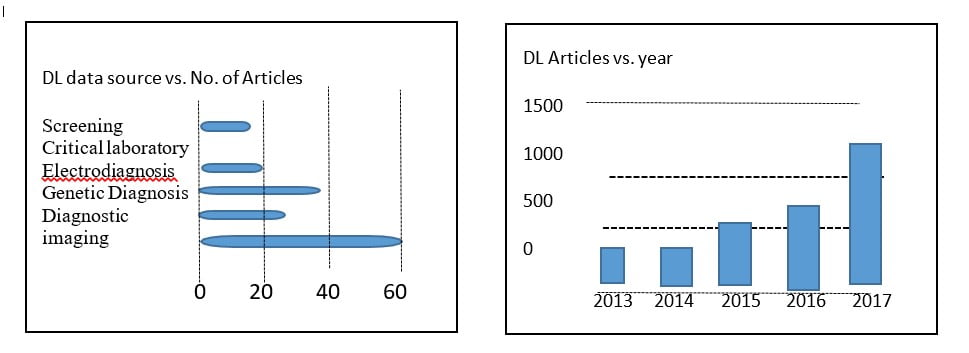
RESEARCH METHODOLOGIES
Introduction
Deep Learning and neural networks are used in this research. Neural networks aim to capture non-linear patterns in data by adding layers of parameters to the model, in contrast to linear and logistic regressions, which are regarded as linear models. The straightforward neural network in the graphic below contains three inputs, one hidden layer with five parameters, and an output layer.
Dimensionality Reduction, Ensemble Methods, Neural Networks, and Deep Learning are further methodologies. Deep Learning and neural networks will be employed in this project. The majority of the information is drawn from studies that were conducted and collected from websites, books on deep learning predictive analytics, journal articles, and scientific journals.
Design Method
This research uses quantitative and qualitative approaches. As it will deal with healthcare and learning relating to illness prediction, diagnosis, and treatment, my attention will be drawn more to the quantitative aspects. I will be dealing with data gathering, forming learning tools, and predicting results in healthcare.
Justification of Method
This approach is acceptable since it applies healthcare predictive analytic techniques such as data profile transformation, sequential pattern analysis, and time series tracks. It makes use of data collection, analysis, and prediction, forecasting outcomes based on patterns seen.
Deep Learning Tools
Deep Learning provides a set of instruments that employ computers to transform data into useful knowledge. A significant component of machine learning is the use of tools, and choosing the appropriate tool can be just as crucial as using the best algorithms.
Tools | Features |
Tensorflow [42] | Using data flow graphs to build models, an open-source artificial intelligence library enables programmers to build massive neural networks with numerous layers. |
MXNet [34] | An open-source software library that uses data flow graphs for numerical calculation and is compatible with DL architectures SNN and RNN |
Caffe [6] | C++, Matlab, and Python programming interfaces with cross-platform support |
Theano \sKeras | provides features like symbolic API support for looping control (scan), which makes it simple and effective to create RNNs. |
The ConvNet [12] | a deep learning library built on Theano |
Using Deeplearning4j [14] | Convolutional neural network toolbox in Matlab |
Singa Apache [2] | a Java and Scala distributed neural network library that is open-source and licensed under the Apache 2.0 standard |
H20.ai [21] | Deep learning library available as open source |
MXNet [34] | Leading healthcare organizations to deploy AI solutions using open source, revolutionizing the sector. |
Deep Learning Existing Framework for Healthcare Predictions
Deep learning uses layers of neural networks and advanced processing capacity to uncover complex patterns in vast volumes of data. The algorithms can handle complex data with a variety of structures thanks to the extension of the standard neural network that employs more hidden layers (Goodfellow, 2016).
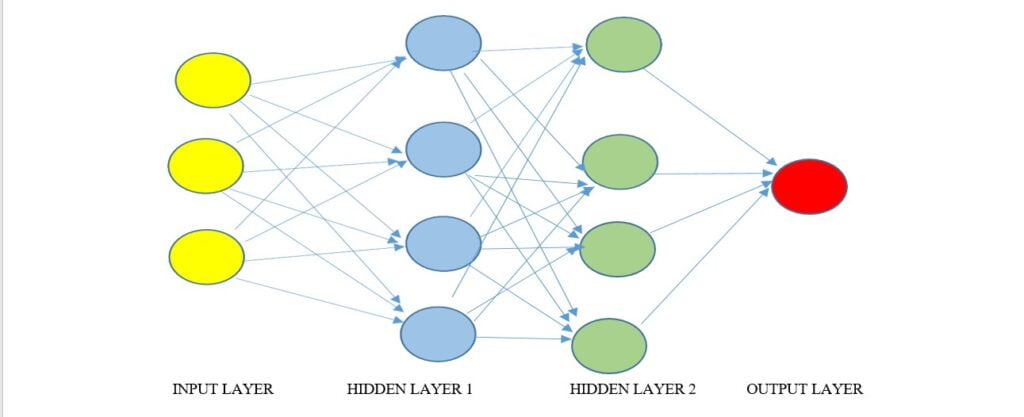
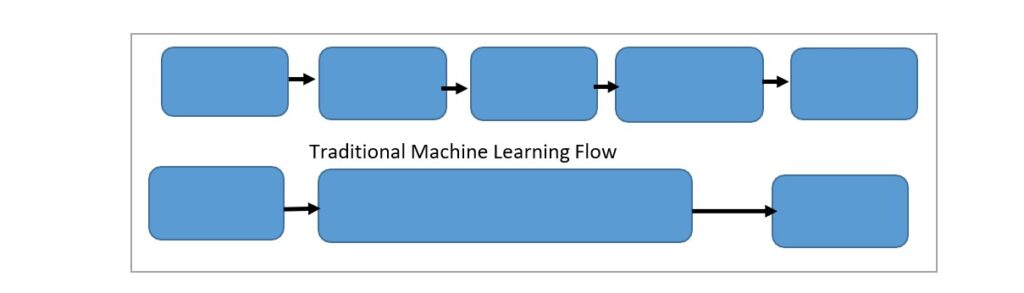
Research Answers
A) What are the deficiencies in the use of traditional machines in diagnosing and treating?
Interview and Clinical History
A doctor can make a diagnosis and develop a close relationship with a patient by asking about their clinical history and talking with them during an interview. The adage “Just listen to your patient; he is telling you the diagnosis” is attributed to William Osler (Gandhi, 2000). A clinician gathers the patient’s medical history during the initial patient interview and verifies the accuracy of the data in the patient’s medical record.
PHYSICAL EXAM
Using your hands, you must observe the patient during the physical examination. Starting with the patient’s demeanor, complexion, posture, level of discomfort, and other characteristics that may help to clarify the health issue, a therapist assesses them (Davies RH, Rees B., 2010). If the practitioner has previously treated the patient, these findings might be contrasted with those from those sessions.
DIAGNOSTIC TESTING
Diagnostic testing has become a crucial component of conventional medical practice throughout the past century (Berger D., 1999); (European Society of Radiology, 2010). Because each cycle of information gathering, integration, and interpretation helps to clarify the working diagnosis, diagnostic testing can be performed in stages. Diagnostic tests are often able to spot issues before they become clinically apparent; for instance, an imaging test that displays coronary artery blockage might spot coronary artery disease even in the absence of symptoms.
B) What does Deep Learning Predictive Analytics provide that eliminates time consumption?
Predictive analytics is unquestionably more important than ever. In his introduction to Predictive Analytics, Second Edition, Oklahoma State University management science and information systems professor Delen stated that “the old notion of learning from mistakes no longer applies” and that “One strike and you are out.” Businesses that use business analytics can frequently not only survive in such a situation but also grow.
Data is essential to business analytics and is increasingly the driving force behind commerce. Data generated and gathered from internal operations and outside sources power all businesses, large and small. For instance, businesses gather data on each stage of the buyer’s journey, monitoring the when, what, how much, and how often clients purchase certain products.
C) Will the use of deep learning automation, without human involvement not lead to hitches in the synthesizing of data and providing relevant information for the treatment of rare illnesses?
It has been asserted that despite ongoing developments, technology is still not complex enough to accurately replicate the human mind. It’s likely that AI will always require input from people to regulate the moral issues involved in leaving judgments to the bits and bytes inside a computer algorithm.
Some experts in science contend that the deep learning system doesn’t need human intervention to learn from mistakes because of the various layers in the neural network. It represents a meager step toward creating an artificial general intelligence system that is capable of autonomous decision-making.
D) Is Africa and the developing world prepared to adapt to this new technology given the limitation of electricity, and skilled staff with the ready knowledge and expertise?
Deep learning is used in healthcare in a few African countries, including Liberia, Burundi, South Africa, Zambia, Rwanda, Tunisia, and Senegal, among others. To illustrate, deep learning is employed in Zambia, a low-income nation, to identify and lower diabetes-related blindness to meet the Sustainable Development Goals by 2030. In a population-based diabetic retinopathy screening program there, deep learning is being used to assess an artificial intelligence (AI) model’s accuracy. The difficulties with healthcare have prevented this from developing fully yet.
E) Given the internet glitches and cyber-attacks occurring all over the world, will there be proof of protection from cyber-attacks?
Recognition of network intrusions is difficult since attacks change constantly as a result of new frameworks, technologies, and applications. 2020 saw a 17% increase in cyberattacks, 77% of which were targeted and focused on stealing credentials and personal information from victims. Organizational attacks primarily seek to steal sensitive user data. These measurements provide an important context for contemporary cyberattack detection and prevention. In addition, the healthcare sector is expanding, and most hospitals are using e-healthcare technologies to quickly address the needs of their patients.
PREDICTIONS FOR FUTURE TRENDS IN DEEP LEARNING IN HEALTHCARE
In the near future, intelligent computers will be able to diagnose illnesses, dispense medication, predict diseases, and suggest treatments. This is the hope of doctors and scientists and manufacturers who believe that deep-learning applications will replace human doctors. Since the beginning of digital photography, deep-learning techniques have been used in medical imaging.
To process the medical information of more patients at the five hospitals, Google DeepMind Health is collaborating with the UK’s National Health Service. Recently, IBM Watson acquired Merge’s healthcare management solution, which includes radiology, cardiology, orthopedics, eye care, and other healthcare disciplines. This acquisition strengthens IBM Watson’s prior $1 billion foray into the imaging industry (Lecun, 1998).
CONCLUSION
To utilize deep learning in healthcare, I give a succinct summary of machine learning and deep learning in this paper as it applies to healthcare data prediction analysis. I reviewed the main disease categories for which DL has been used and highlighted the various healthcare industries that DL has investigated. The major goal of this work is to develop a system for tracking healthcare data using DL and predictive analysis. The proposed model can therefore be utilized to successfully validate the empirical results and emphasize their advantages. I discover a significant region that can be highly helpful for medical imaging and is drawing interest in unsupervised learning by examining recent advancements in deep learning. Moreover, I anticipate deep learning
Developing nations are far away from using deep learning predictive analytics in their healthcare systems due to poor healthcare systems, poor management of resources and funding for the health sector, and lack of current-edge equipment and trained experts to carry out clinical diagnoses and treatment. Brain drain due to low salaries has made qualified and smart medical doctors and nurses flee to greener pastures thus leaving national healthcare systems in shambles.
As more investments are made into deep learning in predictive analytics in healthcare, there will arise more threats from cyber-attacks. Therefore, there is a growing need for more funding for smart cyber-security systems to detect these attacks in advance of their deployment as health matters and a minute’s attack can negatively affect human lives and even lead to wrong decisions being made by experts based on corrupt or false data provided for treatment.
REFERENCES
- Agarwal, R. D. (2014). Big data, data science, and analytics: the opportunity and challenge. Res. 25(3), 443–448. Retrieved October 4, 2021,
- Akshay Kumaar M, Duraimurugan Samiayya, P. M. Durai Raj Vincent, et al. (2022). A Hybrid Framework for Intrusion Detection in Healthcare Systems Using Deep Learning. Frontier in Public Health. doi:https://doi.org/10.3389/fpubh.2021.824898
- Allen B and Thorwarth WT. (2014, December 29). Comments from the American College of Radiology. Washington, DC: 2014. Committee on Diagnostic Error in Health Care.
- Bardhan, I. O. (2014). Predictive analytics for readmission of patients with congestive heart failure.
- Inf. Systems. Res. 26(1), 19-39.
- Berger D. (1999). A brief history of medical diagnosis and the birth of the clinical laboratory. Part 4— Fraud and abuse, managed care, and lab consolidation. Medical Laboratory Observer, 31(12)38-42.
- Berlin L. (2014, 1(1):79–84). Radiologic errors, past, present, and future. Diagnosis. 2014. PubMed, pp. 1(1) 79-84.
- Byass P. (1987).
- https://scholar.google.com/scholar_lookup?author=P+Byass+&publication_year=1987&title=Computers+in+Africa%3A+appropriate+technology%3F&journal=Comput+Bull.&volume=3& pages=17.
- Retrieved from Computer Bull: https://scholar.google.com/scholar_lookup?author=P+Byass+&publication_year=1987&title=Computers+in+Africa%3A+appropriate+technology%3F&journal=Comput+Bull.&volume=3 &pages=17
- CDC. (2008). Laboratory medicine: A national status report. The Levin Group.
- CDC), C. f. (2010). National hospital ambulatory medical care survey. Hyattsville, MD: Ambulatory and Hospital Care Statistics Branch, National Center for Health Statistics;
- Cellscope. (2018, November 15). Retrieved from https://www.cellscope.com/.
- Accessed 15 Nov 2018 Chen, G. T. (2018). Predict effective drug combination by deep belief network and ontology fingerprints. J. Biomed. Inform. 85, 149–154.
- Chen, T. e. (2017). S1 and S2 heart sound recognition using deep neural networks. IEEE Trans.
- Biomed. Eng. 64(2), 372–380.
- Choi, E. S. (2017). Using recurrent neural network models for early detection of heart failure onset. J. Am. Med. Inform. Assoc. 24(2), 361-370.
- D’Agostino, R. V. (2008). General cardiovascular risk profile for use in primary care: the Framingham.
- Circulation 117(6), 743-753.
- Davies RH, Rees B. (2010). Eyeballing” the patient. BMJ-PubMed, 340 (c291).
- Dr. S. Smys. (2019). Journal of Information Technology and Digital World, 01 No. 02, 77-86. doi:https://doi.org/10.36548/jitdw.2019.2.003
- European Society of Radiology. (2010). The future role of radiology in healthcare. Insights into Imaging.; article. European Society of Radiology, pp. 1(1) 2-11.
- Forster D. (1992). Expert Systems in Health for Developing Countries: Practice, Problems, and Potential. International Development Research Centre.
- Gandhi, J. (2000). A life in medicine: 321:1087. In W. OSLER, Book review. BMJ. (p. 1087). NATIONAL INSTITUTE OF HEALTH.
- Gharehbaghi, A. B. (2018). Structural risk evaluation of a deep neural network and a Markov model in extracting medical information from phonocardiography. Stud. Health Technol. Inform 251, 157-160.
- Gibson, E. e. (2018). NiftyNet: a deep-learning platform for medical imaging. Compute. Methods Programs. Biomed. 158, 113-122.
This is great….
We hope to see its manifestation in our current dispensation.
Great research bro, deep learning and AI are very important for the advancement of our healthcare services.
What a thorough research, indeed technology is powerhouse for maintaining more sustainable healthcare system for developing countries such as Liberia.